Home
Perspectives
The data conductor
The data conductor
In order to develop artificial intelligence (AI) for use in medical technology, our research scientists need one thing above all: colossal quantities of all kinds of correct and secure medical data. Ren-Yi Lo, Head of our Big Data Office, is in charge of collecting, preparing, and organizing these data. So, what does all that have to do with music? Learn that and more in part six of our #Futureshaper series.
A "data lake" is, in principle, exactly what the term suggests: a giant "lake" of semi-structured data. Based in Princeton, New Jersey, USA colleague Ren-Yi Lo manages one such data lake together with her international team.
The data lake of the “Digital Technology & Innovation” department that Ren-Yi is responsible for comprises some 1.5 billion data points consisting of clinical images, reports and, additionally, billions of laboratory diagnostic data. "If I say these numbers out loud, I, too, find them unfathomable," she admits with a laugh. This truly is "big data"1.
What is big data?
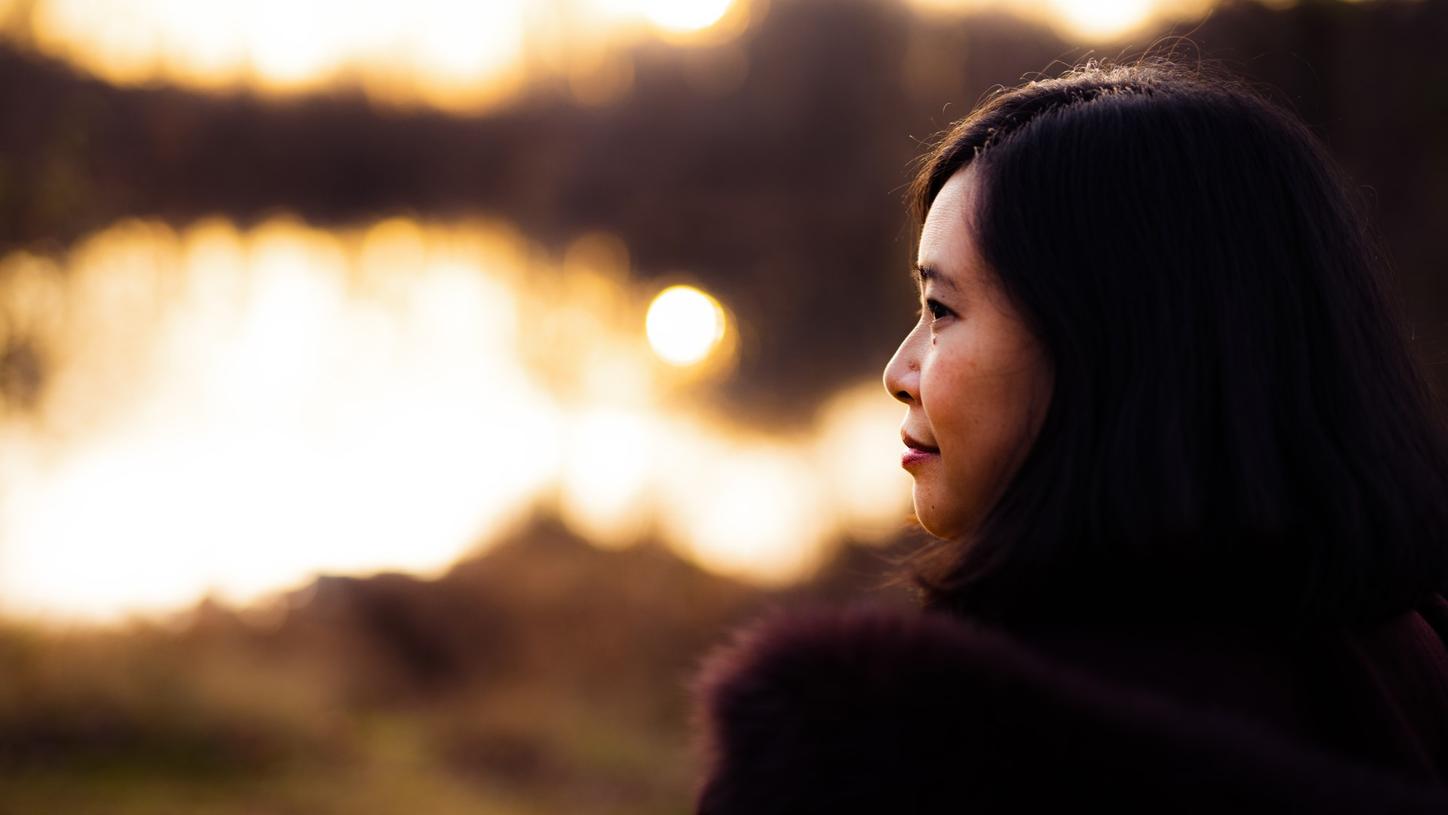
Valuable clinical data can be transformed into high-tech innovations for healthcare: they serve the approximately 250 colleagues in the AI team working at various global locations for the Siemens Healthineers “Digital Technology & Innovation” department as a basis for developing artificial intelligence2 for new applications in the medical field.
AI is a crucial pillar for the future of medical technology: For example, it can automate routine processes along the clinical pathway, and thereby ease the burden on healthcare professionals in their daily work.
What is artificial intelligence (AI)?
The quality of the data is decisive
Artificial intelligence is based on algorithms that have been trained with a large quantity of anonymized and
curated3 data from the data lake. The algorithms cannot be used in clinical routines until they have undergone this training.
The quantity and quality of the data that have been used for training and validation are decisive: The larger the quantity of high-quality data, the more successful the efforts are to prevent distortion, and the more precisely the trained computing model will work.
What does data curation mean?
Big data: Invisible, yet essential
As head of our Big Data Office, Ren-Yi Lo and team ensure that these enormous quantities of data are collected, prepared, and managed. This work, while done for the most part unseen in the background, is so vital. Metaphorically speaking, one could say that Ren-Yi and her team are in charge of filling the "data lake". They prevent the lake from "running over" in an uncontrolled manner, and ensure that no content “drowns” in the lake.
Become part of the Siemens Healthineers team
Are you also interested in joining our enthusiastic team?
I sometimes feel a bit like I'm a 'Jill of all trades*. But I suppose that's really exactly what I have to be for my job.
Ren-Yi Lo
Head of Big Data Office, Siemens Healthineers
* In other words, a woman who is proficient in very many fields, without possessing absolutely complete expertise in any one field.
Ren-Yi, whose parents have Chinese roots and have lived in Germany and the United States, studied computer science with a particular focus on systems engineering. "My work today as Head of the Big Data Office is substantially more varied than my university studies," notes the 40-year-old with a smile: "My job, actually, is to solve complex problems."
For that to succeed, she has to speak the languages of multiple professional fields in order to coordinate various interest groups: "Medicalese" with clinical partners from whom the data originates, "Technicalese" and "Software Shop Talk" with AI research groups, developers, and her team from the Big Data Office, and "Legalese" with colleagues from the Data Protection and Privacy, Legal, or Intellectual Property Departments.
- Data are used by researchers in the field of AI to train algorithms that are of fundamental importance to our future technologies. When AI scientists invent something for improving the training methods used or the algorithms themselves, then such inventions can also be protected by patents. At Siemens Healthineers, a team of patent attorneys from Intellectual Property Department work closely with our AI scientists to identify valuable discoveries and inventions and strategically protect them against plagiarists. Siemens Healthineers holds some 23,000 intellectual property rights, of which over 15,000 are granted patents.
What data do AI scientists need for their research work? "That can vary extensively, and it depends on the given research project," Ren-Yi explains. For example, AI researchers are currently working on a project that aims to optimize laboratory diagnostics with the aid of artificial intelligence.
How can AI optimize laboratory workflows?
Another project is conducting research aimed at creating a digital twin of the human liver. When the research team's work kicked off, they came to Ren-Yi to jointly define with her what data they would need and where such data could potentially be sourced from. There are countless types of data. In a nutshell, five different types of data are used at Siemens Healthineers for training AI algorithms:
How do you generate a computer model of the human liver?
Senior AI research scientist Chloé Audigier is conducting research aimed at creating a digital twin of the human liver. Such models can help physicians simulate multiple treatment options.
Data from medical imaging include, for example, radiographic (X-ray) images, computed tomography (CT) scans, magnetic resonance imaging (MRI), and ultrasonic images. Accompanying medical reports contain background information on the medical history of patients and the treatments administered. Data from laboratory diagnostics comprise, for example, results from laboratory testing of blood, urine, and body tissue. Genomic data are data derived from DNA analyses. Operational data contain information on operational workflows in physicians' practices or hospitals, as well as on maintenance work performed on medical devices, etc.
These various types of data must later be collected in the data lake in an organized structure and stored to be easily retrievable.
The right data cohorts
Another challenge for Ren-Yi and her team is that, in order to develop trustworthy, reliable AI algorithms, the data made available for testing must originate from fitting cohorts4: "This means that, in our data acquisition, we need a balanced cross-section of people of differing genders, ages, and ethnicities; healthy people and those suffering illnesses, and so forth." The data used for each project must represent the statistics of the population and problem for which the subsequent AI systems are intended. "Otherwise, there could be a bias within the AI," explains Ren-Yi, a distortion or deviation from reality in the results: "It must be our goal to prevent inequity within the AI."
And where do these data come from? "We collaborate with a network of some 175 partners around the globe to procure the fitting data," says Ren-Yi, with a touch of pride in her voice. These partners include many renowned medical centers, hospitals and university clinics.
What is a cohort?
Complex standardized process
Before any data may be used for AI research projects, they must undergo a complex standardized process to ensure the highest possible data security. After all, we work here with the data subjects' most personal information of all, a fact that Ren-Yi is keenly aware of: "Every single data point we use for AI training originates from patients. And patients are individuals whose rights we absolutely must protect."
Process overview of the data lifecycle
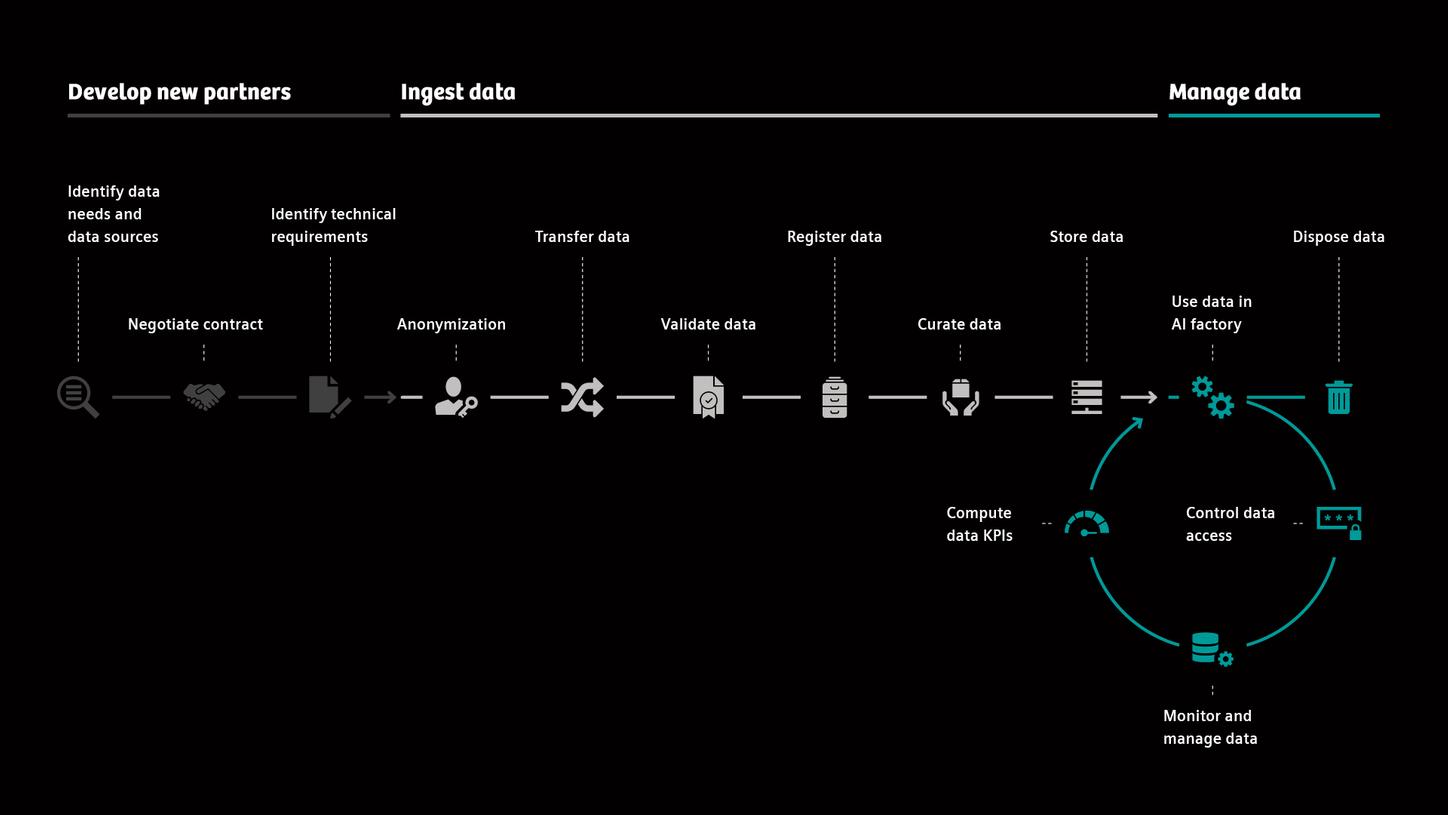
This is why detailed contractual agreements are concluded with each data supplier before any data are transferred to us, contracts whose content Ren-Yi jointly develops with colleagues from the Legal department and collaboration managers of the respective business units. These agreements contain information like: What statutory regulations govern the data storage and processing, such as the requirements defined in the General Data Protection Regulation (GDPR)5? How exactly are the data to be used and stored? Who exactly is permitted to use the data?
"All of that is regulated on an entirely individual basis." When data are registered, Ren-Yi and her team ensure with the aid of a sophisticated digital tool landscape that only the contractually defined group of persons and projects have access to the given data pool.
What is the GDPR?
Anonymization is essential
For reasons of data protection and privacy, data are anonymized at the respective data source before the secure transfer to the data lake can take place. This means that any information enabling direct inference to the data subject, e.g., the person from whom the data originate, are removed. "For example, the data supplier erases all names, dates of birth and addresses of the data subjects. This information isn't relevant for our AI training purposes anyway," explains Ren-Yi.
Once received, the team validates the anonymized data with specialized tools and applies the four-eye principle for verification. This means that the data are reviewed for various quality aspects:
It is also of key importance to correctly index the data within the framework of data curation and administration, e.g. the sensible and useful "filing" of the data. For example, specific search criteria like keywords are attached to the data. This ensures they remain retrievable at any time in case, for example, they're later required to undergo auditing by governmental authorities such as the United States Food and Drug Administration (FDA)6.
If a contractual partner subsequently revokes its consent to use its data, or if a patient does the same, or if the data are simply no longer needed, it must be technically feasible to "fish" the precise data set out of the data lake and erase it.
What is the FDA?
Sherlock, the AI supercomputer
So, where are the data physically stored? The Digital Technology & Innovation Department where Ren-Yi works in Princeton has built its own supercomputing infrastructure in which the data for the data lake are managed together with the AI training: called the Sherlock supercomputer. It's one of the most powerful supercomputers dedicated to developing AI in the medical technology sector.
In its current configuration, Sherlock sports a processing speed of 100 petaflops. This means it can perform 100 times a quadrillion computing operations – per second. "Yet another mind-blowing number," laughs Ren-Yi.
And the huge numbers just keep coming: The data lake currently has a storage capacity of one petabyte, while the Sherlock supercomputing platform is equipped with over 13 petabytes of memory capacity. One petabyte corresponds to 1,024 terabytes. Here, too, the Big Data Office fulfills an important mission in regards to cybersecurity and system failure management: "We ensure that, at any given time, we have backups and disaster recovery plans in place for the data in our data lake," explains Ren-Yi.
With the data from the data lake and the supercomputing power of Sherlock, our researchers can perform some 1,200 AI experiments every day. Ren-Yi is proud of the contribution that she and her team have made in the field of data management:
What is a terabyte?
In future, AI will play an indispensable role in daily clinical routines, translating the growing data quantities into the knowledge needed to make the right decisions. Today, for example, the AI RAD Companion – our family of AI-supported workflow solutions – can ease the burden on medical personnel in their daily routines.
Want to read more about the AI RAD Companion?
The big opportunities of digital models
Digital models developed using AI can help to better understand the health status of individuals to predict changes and plan treatment options on a more personalized basis – thereby improving treatment results.
Leveraging this potential requires people like Ren-Yi and her team, who are mostly unseen and in the background, to "conduct" and direct valuable data that need to be protected – and make AI and the associated research possible in the first place.
© Photos: Markus Ulbrich
© Video: Markus Ulbrich (direction, camera); Cagdas Cubuk (camera, sound);
Lisa Fiedler (editing); Katja Gäbelein (concept)
© Motion Graphics: Viola Wolfermann
© Graphics: Stefanie Schubert, Bianca Schmitt
Share this page
Katja Gäbelein works as an editor in corporate communications at Siemens Healthineers, and specializes in technology and innovation topics. She writes for text and film media.
Assistant editor: Guadalupe Sanchez
- Sources
Al-Mekhlal, Monerah; Khwaja, Amir Ali (2019): A Synthesis of Big Data Definition and Characteristics. In: IEEE International Conference on Computational Science and Engineering (CSE) and IEEE International Conference on Embedded and Ubiquitous Computing (EUC). pp. 314-322. Available online: https://ieeexplore.ieee.org/abstract/document/8919591
Gethmann, Carl Friedrich; Buxmann, Peter; Distelrah, Julia; Humm, Bernhard G.; Lingner, Stephan; Nitsch, Verena; Schmidt, Jan C.; Spiecker (Döhmann), Indra (2022): Künstliche Intelligenz in der Forschung – Neue Möglichkeiten und Herausforderungen für die Wissenschaft. (Artificial intelligence in research – New opportunities and challenges for science) Berlin: Springer, p. 8.
- Disclaimer
- The presented information is based on research results that are not commercially available. Its future availability cannot be ensured.
- The figures shown here are as of February 2023.