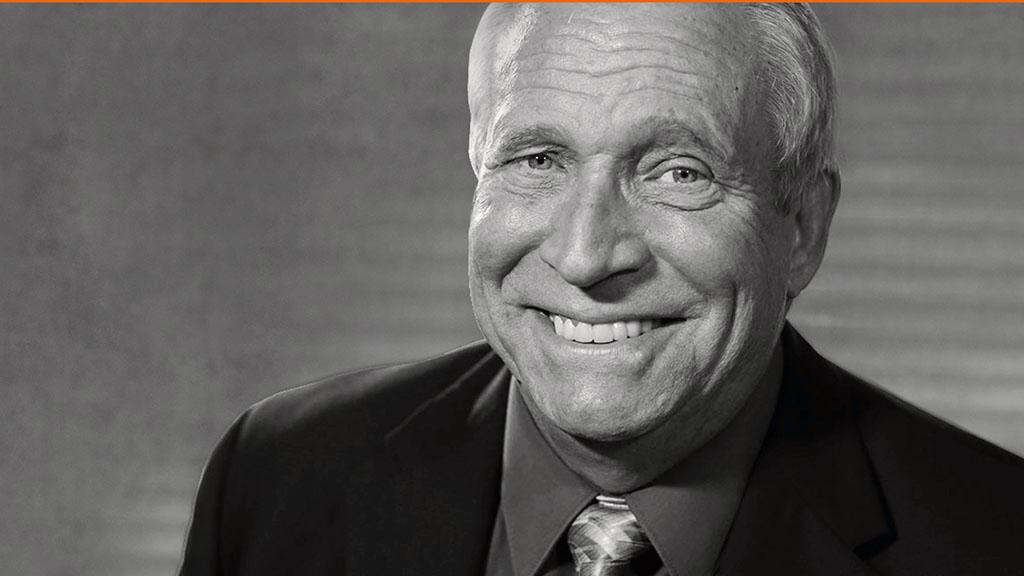
The future will always be just that…until it becomes the present. That is especially true when the future involves science. What if science fiction has a way of turning – suddenly – into science fact. Like flying machines and spacecraft. One day they were theoretical. The next they were happening.
At the annual meeting of the Healthcare Information and Management Systems Society, or HIMSS, in February, companies told me how they had for years been building smart algorithms. One called them the secret sauce of automation. Their software, they told me, had been running for more than a decade on the graphical processing units, or GPUs, that are now recognized as indispensable to artificially intelligent machines.
The “term du jour”
Only in the last couple years has it been OK for these companies to say they were building artificial intelligence, widely known simply as AI, into their machines. Not because AI is scary, although fear is one of the emotions stirred by thinking machines. But because it would not have been credible to say so. That has changed. Today, if you want your company to be seen as a technology leader, you’d better at least be looking into AI. AI has become the term du jour. But it won’t be for long. Why? Because the term is steeped in hype. Make no mistake. Artificial intelligence will continue, but under a different name. Many equipment developers are already opting for the more precise term “Machine Learning,” or ML. This denotes the processing of data in decidedly nonhuman ways. Typically, ML is used when the technology is being applied not as an end but as a means to something greater. Machine Learning is part of automation, which itself has been used for many years by developers of imaging equipment. As such, it is the way to achieve this cornerstone of value-based medicine with its trifecta of raison d'etres – efficiency, cost effectiveness and patient benefit.
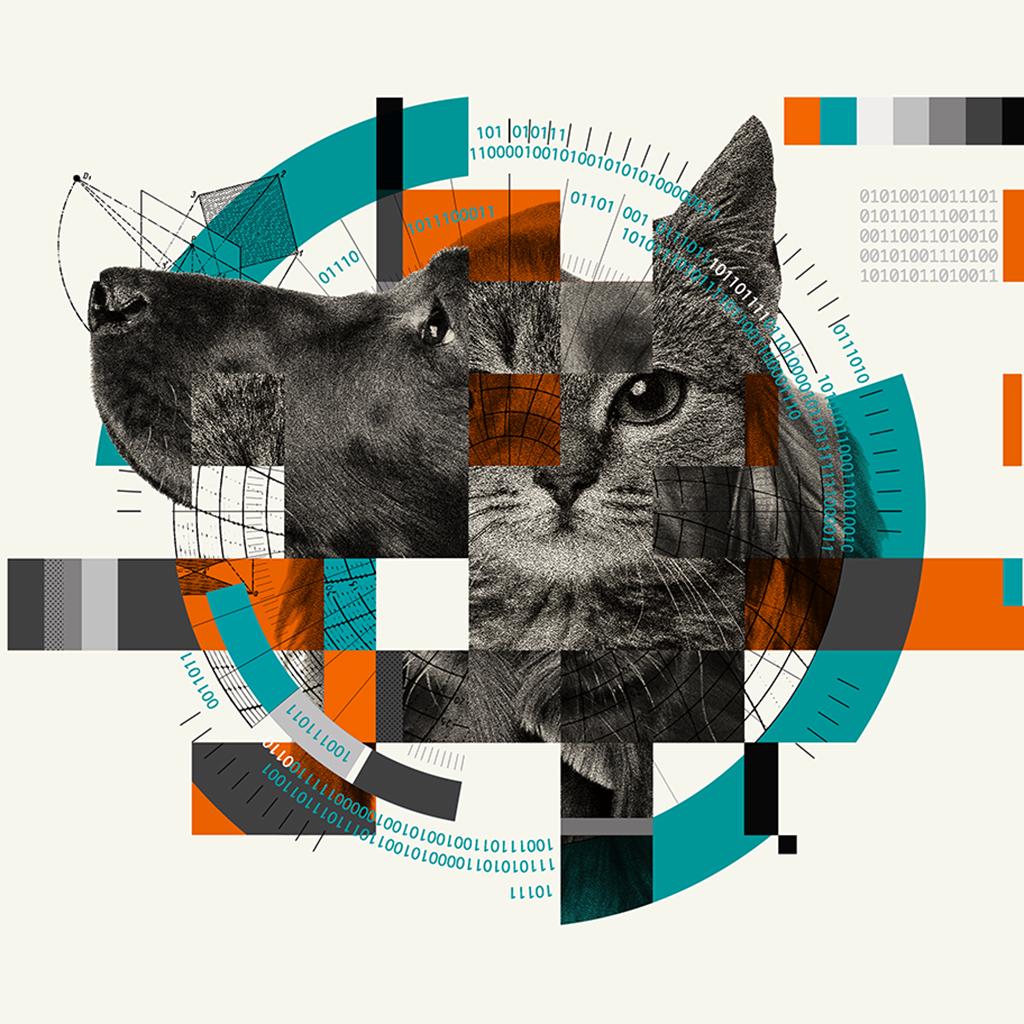
How Machines Learn
Through a process called deep learning, algorithms discovered what distinguishes a dog from a cat. In the world of medical imaging, they are learning to distinguish healthy from abnormal…what scan slices to load for a physician to make a diagnosis…how to position a patient to get the best image with the least radiation.
Because unsupervised deep learning allows machines to figure out the rules on their own, they might “see” things that people don’t. This has given some people pause. Questions arise as to whether physicians or patients should trust them. But we’ve gone down a similar path before.
I used to tune my own car using a strobe light and a timing chain. I couldn’t do that with modern cars, which must be taken to mechanics who tune them using computers. Yet these cars go further between tune-ups; get better mileage; cost less to run; and make us safer. In other words, we have learned to have faith in the machines we create.
But – importantly – the goals behind machine learning must be determined by people. People – whether they are physicians or patients – must hold the reins.
With the ability to learn, machines can do what humans cannot – they can make sense of volumes of data so enormous, so complex, that humans have no chance of understanding them. From machine learning may come ways to achieve efficiencies and solutions that people alone could not fathom. But – importantly – the goals behind machine learning must be determined by people. People – whether they are physicians or patients – must hold the reins. Under such a condition, intelligent machines would not, by their own volition, present an existential risk to humanity. It won’t matter how much data intelligent machines process or how fast they do it. They will be directed to apply their incredible processing power to do what people cannot.
Why Docs And Patients Have To Be On Their Toes
Like cars that go many times faster than humans can run; like airplanes that travel many times faster than cars; thinking machines will think of things that are beyond the capability of people. And, like cars and airplanes, they will sometimes crash. But, if past experience is a guide, designers will become more careful in their direction. And the flaws will be fixed. Thinking machines will continue to think – and they will do so more wisely.Physicians should not be asked to – nor should they ever – blindly accept the conclusions of thinking machines. But they must know enough about smart machines to spot the signs of trouble – and know when adjustments are needed.
About the Author
Greg Freiherr is a contributing editor to Imaging Technology News, or ITN. Over the past three decades, he has served as a business and technology editor for publications in medical imaging, as well as a consultant to industry, academia, and financial institutions.