A team of radiologists and IT experts at the University Hospital Basel, Switzerland, are working with Siemens Healthineers to develop AI solutions for the hospitals of tomorrow. The idea is to use algorithms throughout the clinical diagnostic process – and enable diagnostics to keep up with the constantly growing demands it is facing.
Photos: Philip Frowein
The University Hospital Basel is an impressive complex located near Basel’s historic city center. A few steps further, directly on the picturesque Rhine river, several clinical research groups share a building. Patients don’t usually come to this location, yet thousands of digital patient records move around here all the time. On the ground floor, four radiologists, five data scientists, and one pathologist sit facing two dozen large screens as they work on the algorithm-based future of clinical diagnostics.
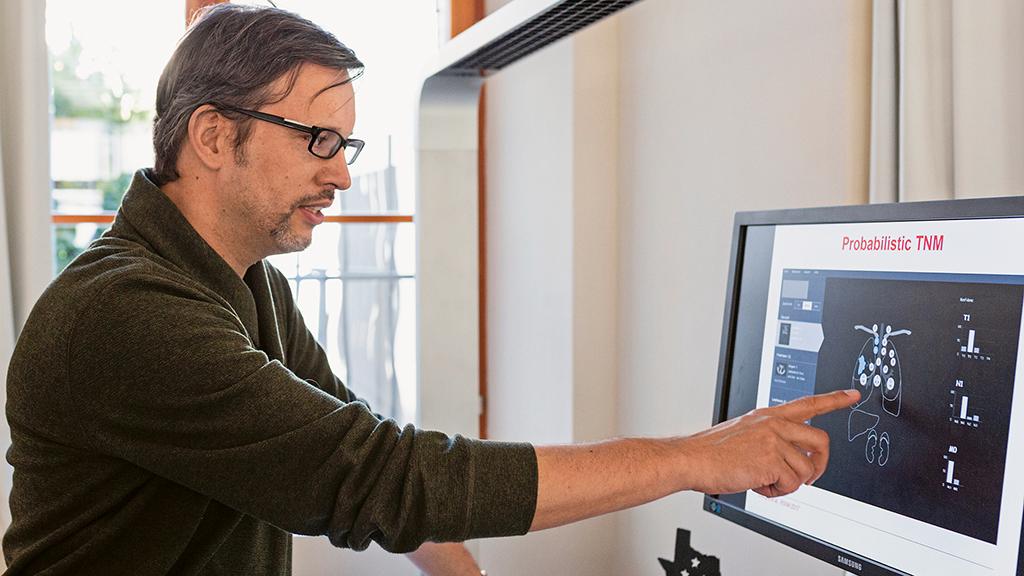
Algorithms for More Accurate Biopsies
Bram Stieltjes, MD, PhD, himself a radiologist, leads the working group. He is not only Head of Radiology Research at the University Hospital Basel, but was recently made Head of IT Research, too. So he is kept busy. Just recently, one of Stieltjes’ employees left for a year at Siemens Healthineers research center in Princeton, USA – one of the most important centers for artificial intelligence (AI) and machine learning in radiology. The collaboration with Princeton could give impetus to many ambitious AI research and development projects, including one that is particularly close to Stieltjes’ heart at the moment.
The project relates to prostate cancer patients facing biopsy. The vision is that magnetic resonance imaging (MRI) data sets of the prostate will be automatically segmented using machine learning and that the algorithms will then help indicate the best site for a biopsy. This information will not end up in a medical report, but will be directly transmitted to an ultrasound device in the form of an annotated image. “The doctor who makes the ultrasound guided biopsy no longer has to deal with different images and findings,” says Stieltjes. “He or she has all the necessary information on the image itself, which facilitates an accurate biopsy.”
The prostate project, which is still in the early planning stage, is so exciting for Stieltjes, because it shows how a complete clinical diagnostic process involving different imaging modalities could be digitally integrated with the help of machine learning algorithms: “I think this is the direction we should be going. Clinical care will gain most from this approach.”
A hospital cannot handle development projects like this alone. In addition to academic partners, it also requires close cooperation with companies offering hardware and software solutions for clinical diagnostics. Siemens Healthineers is a key cooperation partner of Stieltjes and his team. “The core idea of our group is to be a bridge connecting industry and clinical and academic research. We can develop prototypes relatively fast and test them in a clinic-like environment.”
Need to Improve Data Preparation and IT Integration
A good example of the possibilities, but also the difficulties, of using AI in diagnostics is a prototype AI system for X-ray and computed tomography (CT) examinations developed by the University Hospital Basel. The aim is to ensure a quick diagnosis for those patients requiring it, such as those with fractures or bleeding. “We have two problems,” says Stieltjes. “Three out of ten patients without clear symptoms have acute pathology. And, conversely, nearly half of the patients classified as priority cases by clinicians turn out not to be urgent cases after all.”
The Basel team has therefore trained an algorithm to differentiate acute cases from normal findings and to prioritize patients on the radiology worklist accordingly. “It works extremely well from both a technical and a clinical perspective, and our clinicians are enthusiastic,” says Stieltjes. However, integrating it into the clinical processes proved difficult, not owing to the algorithm, but to the available IT infrastructure.
“Unfortunately, because of the way we process clinical data in radiology, we are not really ready yet for self-learning algorithms. There is still a lot that needs to change.” As an example, Stieltjes says that it should be considered whether there will still be a written diagnostic report in the future or whether radiologists should rather just annotate the images. For algorithms that would be much more practical. And it would probably make no difference to the clinicians.
We have two problems: Three out of ten patients without clear symptoms have acute pathology. And, conversely, nearly half of the patients classified as priority cases by clinicians turn out not to be urgent cases after all.
Sophisticated Support for Thoracic Examinations
The Siemens Healthineers AI-Rad Companion1, which was launched at the 2018 convention of the North American Radiological Society (RSNA) in Chicago, has successfully passed the prototype stage. Bram Stieltjes and the Basel working group continue to be closely involved in the ongoing development of this platform.
The tool aims to support radiologists in their diagnosis – at present only with CT images of the thorax. The platform may take pressure off the radiologist, and optimize the quality and consistency of their diagnostics. Overall, Stieltjes considers the algorithms that have been developed to be very well engineered. However, relatively large amounts of training data are needed if the algorithms are also to cope with patients who have more unusual anatomies or findings. “You notice how the systems were trained,” says Stieltjes. Normal or slightly pathological findings are commonly over-represented. So, often the results of validation studies cannot be transferred directly to clinical collectives.
However, Stieltjes emphasizes that it is by no means always the algorithms that are wrong: “When radiologists create a report, there is a variability of about ten to 20 percent depending on the examiner. In an algorithm-based diagnosis, this variability is completely eliminated and the results are very consistent. That alone is a huge advantage of AI platforms like the AI-Rad Companion,” says Stieltjes.
When radiologists create a report, there is a variability of about ten to 20 percent depending on the examiner. In an algorithm-based diagnosis, this variability is completely eliminated and the results are very consistent.
Algorithms for Integrated Decision Support
Another Siemens Healthineers application that the Basel group is currently evaluating at the prototype stage is the AI-Pathway Companion2. “This application is about integrated decision support,” says Stieltjes. It aggregates and analyzes patient’s longitudinal data, along with results from imaging, laboratory, pathology and clinical studies. Using this as the basis, the system automatically map’s the patient’s status in the clinical pathway, taking evidence-based clinical guidelines into consideration, and recommends the next steps.
AI algorithms are also used to quantify risks and to predict the individual course of disease. In cases of prostate cancer3, for instance, the software supports the work of the tumor boards and facilitates interdisciplinary collaboration. “Clinically, the ultimate goal is to decide about the most appropriate treatment for the individual patient,” says Stieltjes. But the software can also be used for process optimization, he says, for example by analyzing how many diagnostic steps were needed to reach a particular decision.
“In five to ten years, there will be hardly any diagnostic processes that are not supported by AI.
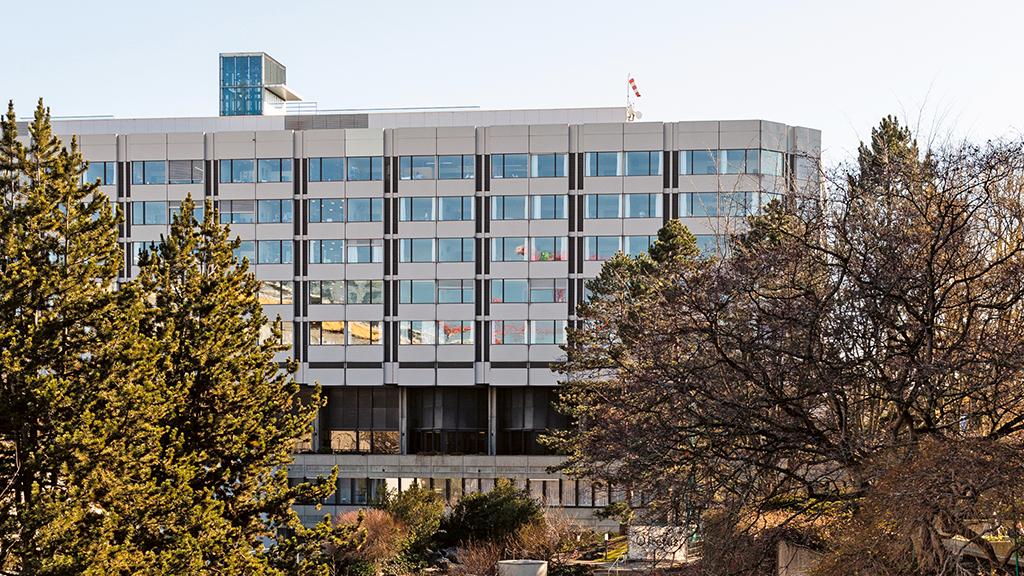
Driver for Digitalization
All in all, Stieltjes is optimistic that it will soon no longer be a case of individual radiology departments using individual AI applications for very specific purposes: “In five to ten years, there will be hardly any diagnostic processes that are not supported by AI.” He says one of the factors behind this will be the growing pressure on the diagnostic fields as a result of ever-increasing and more extensive examinations and data sets: “The data volume in medicine doubles every two to three years. That's a huge driver for digitalization.”
Stieltjes refers to a study from the US, which found that 50,000 new diagnostic positions would have to be filled in the national healthcare system over the next ten years to meet growing demand: “With human resources alone we cannot meet these challenges. If we don’t change our processes and use more AI algorithms, diagnostic quality will decrease. We need new tools to deal with the flood of data we can expect.”
About The Author
Philipp Grätzel von Grätz is a medical doctor turned freelance writer and book author based in Berlin, Germany.