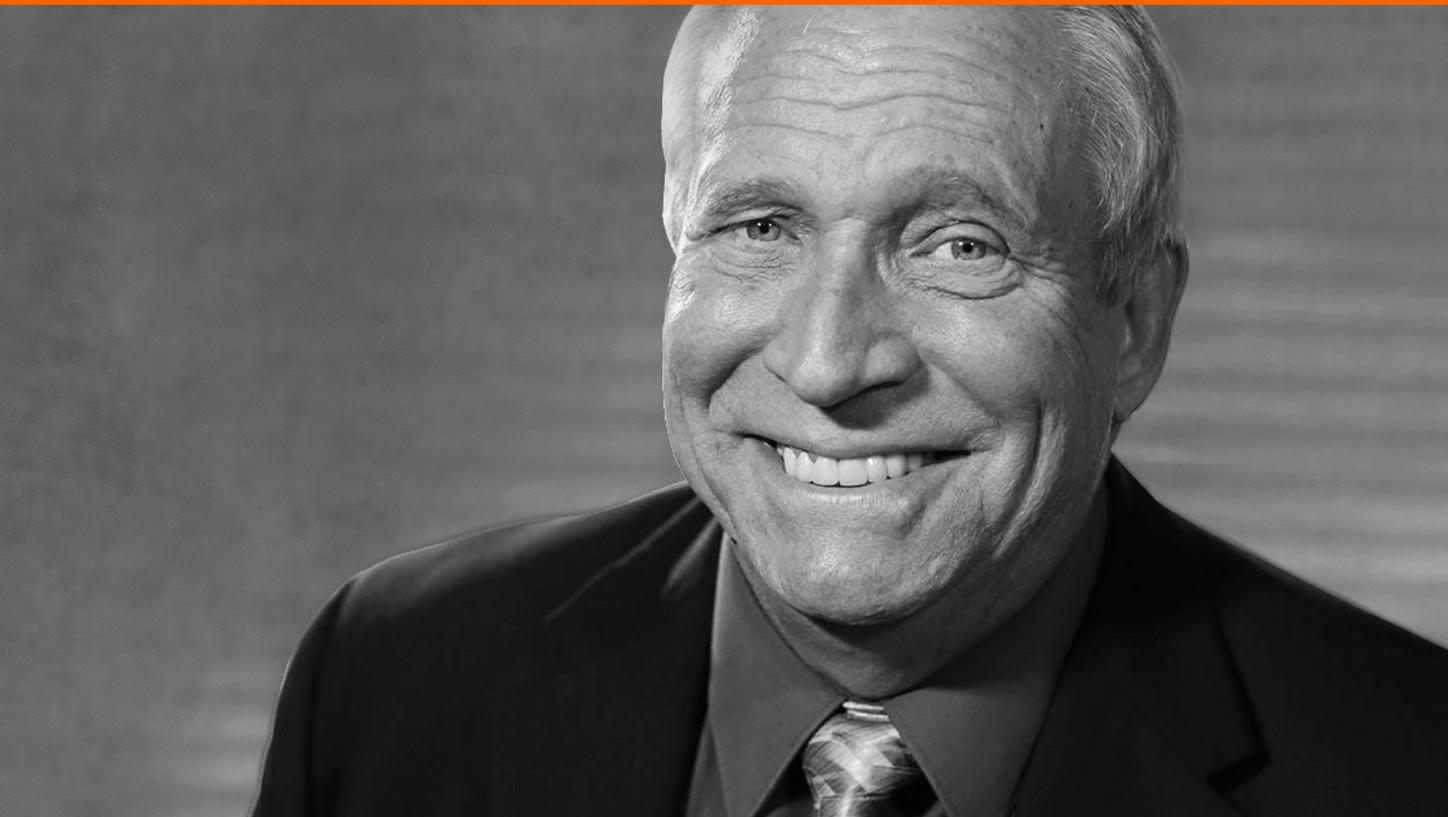
You won’t find many doctors who went to medical school to become data clerks. But that’s what many have become. Sometimes with their backs to patients, they sit in front of a computer screen, typing and clicking. Some may try to carry on conversations with their patients as they input data. But it’s tough. Technology has gotten in the way of the doctor-patient bond. Artificial intelligence might help get rid of that barrier.
Where Natural Language Processing (NLP) Fits
Smart algorithms – fueled with natural language processing – are being groomed to recognize doctors’ questions and patients’ answers; extract key points and put the information into electronic health records. Doing so might save doctors between a third and half of their workdays, possibly reducing burnout and giving doctors more time with patients.
The key word, of course, is “might.”
The future of medical AI is highly speculative. But in some cases, the speculation may be warranted.
Much depends on the development of NLP, an enabler of medical artificial intelligence (AI). For years it has been known that algorithms capable of processing written language can extract laboratory test data, even from unstructured clinical notes. Similar potential exists in the doctor’s office.
I have seen an NLP-based system that listens to doctor-patient exchanges; translates what was said into text; extracts key words and phrases; then plugs them into a structured medical report. Strategically placed microphones pick up the voices of doctor and patient; smart algorithms do the rest.
This barely hints at how NLP might change health care for the better. In late April 2019, researchers described their development of an NLP-based system that translates human thoughts into a synthetic voice. In this research, described in a paper published April 24 in the journal Nature, an electronic sensor implanted onto a human brain picks up signals meant to produce speech. An AI algorithm translates these signals into a synthetic voice.[1]
If commercialized, the technology might give voice to patients who cannot speak for themselves – victims of stroke, traumatic brain injury or neurodegenerative diseases, such as Parkinson’s, multiple sclerosis or amyotrophic lateral sclerosis (Lou Gehrig’s disease). But such technology could be a long time away. Maybe a decade or more. A lot of development needs to be done.
In the nearer future, smart algorithms might help patients make sense of what they access through patient portals.
Why AI Might Soon Improve Healthcare
In the nearer future, smart algorithms might help patients make sense of what they access through patient portals – conduits for transferring medical information to patients. The potential of NLP to do this has already been demonstrated. So has the potential to make technical explanations understandable by the public.
A “chatbot” called Bold360ai is being sold to businesses to interpret complex language for their customers. As Bold360ai reportedly holds textual conversations, it “remembers” context. Is it farfetched, therefore, to believe that similarly chatty AI algorithms might interpret medical language in context?
Chatbots like this could have an enormous effect. Imagine doctors concentrating just on patients’ most complex questions; and patients thoroughly understanding their medical information.
And NLP might be extended even further – to patient questionnaires, like the ones that patients now fill out routinely in waiting rooms.
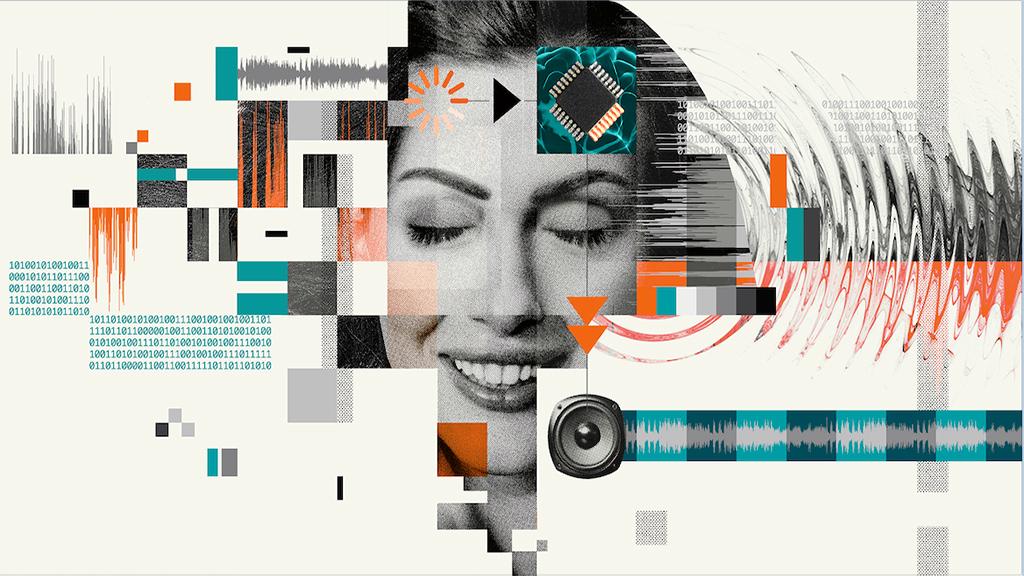
Individualizing Health Care
What if smart algorithms turned the data in patient questionnaires into tailor-made healthcare strategies? A screening mammography strategy, individualized for a specific woman, might be based on all the risk factors that determine her vulnerability to breast cancer. Age, currently the sole basis for mammography screening guidelines, might be considered along with family history. Smart algorithms might pluck breast density and past biopsy results from the patient’s electronic record; calculate the benefit/harm ratio for a specific patient to have a screening mammogram annually or biannually; and put the findings into a strategy for the doctor and patient to discuss.
What if similar strategies could be developed for patients at risk of developing lung cancer? Diabetes? Heart disease? Already a company is developing an NLP text mining platform that digs into medical risk factors, monitors patients for these risks, measures quality of care, even improves patients’ clinical documentation.
Taking this a step further, could AI provide the scientific basis for factors whose role in disease now is only suspected? Among them: “food insecurity” – how many times have we heard news reports about people who must choose between medication and food? – mental health and substance abuse.
Helping Doctors Get Back To Their Roots
In the meantime, AI might be built into “virtual medical assistants,” processing data about patient interactions in the context of medical literature to help physicians apply clinical guidelines; monitor the quality of care they give; predict adverse drug events; even identify rare diseases.
If these possibilities turn into realities, physicians might be able to do what led them to medical school in the first place.
About the Author
Greg Freiherr is a contributing editor to Imaging Technology News, or ITN. Over the past three decades, he has served as a business and technology editor for publications in medical imaging, as well as a consultant to industry, academia, and financial institutions.